Integrating data from multiple sources that employ different structures and schema has always posed complex, messy problems for IT professionals. Today’s growing volume of data and data types made things even more complicated. Here are some key tips to help your organization integrate its increasing amounts of data.
Best Friends: Data Integration And Application Integration
Data integration and application integration have traditionally been treated as separate efforts, but change is underway, according to Brian Hopkins, VP and principal analyst serving enterprise architecture professionals at Forrester Research. He cites some pioneering vendors that are building data integration into business process flows. “Big data processing and cheap memory make it possible to store data in its raw or nearly raw format and do complex integration operations on it in memory and just in time,” Hopkins wrote in a recent data integration report. Leveraging this new architecture can be less painful than the data integration effort necessary to create traditional data warehouses and even data lakes.
Applications Vs. Data: Which One Has Authority?
Gartner VP and distinguished analyst Mark Beyer agrees that data integration and application integration are tightly linked and becoming even more so. As we go farther down this path, enterprises need to choose which one has the authority. “They are both trying to manage the data,” he told InformationWeek in an interview. “So there has to be a decision somewhere between the two. Which one has authority over the data?”
Treat Data-Moving Tech As Middleware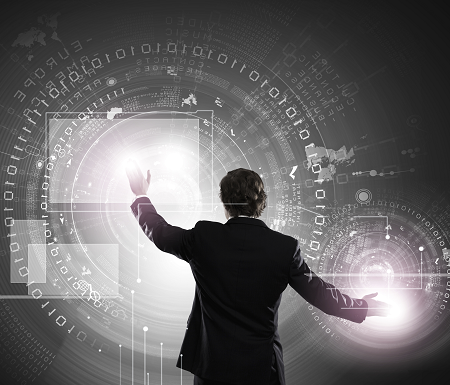
Some people think of moving data from one system to another as a bane or evil that should be avoided at all costs, according to Girish Pancha, CEO of StreamSets. But the emergence of big data makes it impossible not to move the data. He suggests that data architecture pros should think of the technology that’s moving the data as “middleware that needs to be decoupled from all data sources and data stores.” This approach simplifies and speeds up upgrades.
Invest In Modern Architecture
Forrester’s Brian Hopkins told InformationWeek in an interview that enterprises need a modern architecture in order to pursue a successful data integration strategy. Past practices and architectures included ETLs into data warehouses and loading data into data lakes. “The really interesting place where things are changing now is in the application of open source big data tools to manage the bigness and fastness of data in motion at its source… Being able to tap into data streams is a big part of a successful data integration strategy.”
Watch Your Security
When it comes to big data integration, security remains an immature service. Forrester’s Hopkins said this is a big problem in the Hadoop world, because Hadoop distributors Cloudera and Hortonworks are going in different directions with the security of their big data applications suites. “That’s not good for anybody,” he said. So enterprises need to keep a close eye on the security of their data when using these tools.
Let Go Of Control
Gartner’s Beyer said that one big complication for today’s IT pros who are in charge of data infrastructure and data management is dealing with the fact that they are no longer in total control. “The data management model is going to be everywhere. It’s distributed. It’s in the cloud, it’s on-premises, it’s in apps. When you have to do data integration, recognize that the governance model is separate from the management approach. Governance is what you have to do and management is how you have to do it.”
Revisit Metadata
Metadata today is being revisited, according to Gartner’s Beyer, but not so much for its traditional use as a container of static information about the data’s source and value. The new excitement around metadata is focused on how often that data is accessed and used, which then tells you how important that data is. Is the data used in many different types of analysis? Is it regulatory, transactional, or operational? These indicators could tell you that this data is important and requires really good governance.
Look At Content Form And Context Bias
Gartner’s Beyer says he believes that, in the next three to five years, organizations will recognize that data integration and data analysis come with an inherent bias. “In simple terms, ALL data is biased toward the creator,” he wrote in a blog post covering this topic. “All data is captured from multiple perspectives and represents multiple points of bias. That means each new data point reflects the intent of the business process designer. This means it is not possible to actually assemble new analytics from existing data.” Beyer’s solution to this is engaging real data scientists who build out competing interpretations of data. It compares the resulting theories along at least two axes.